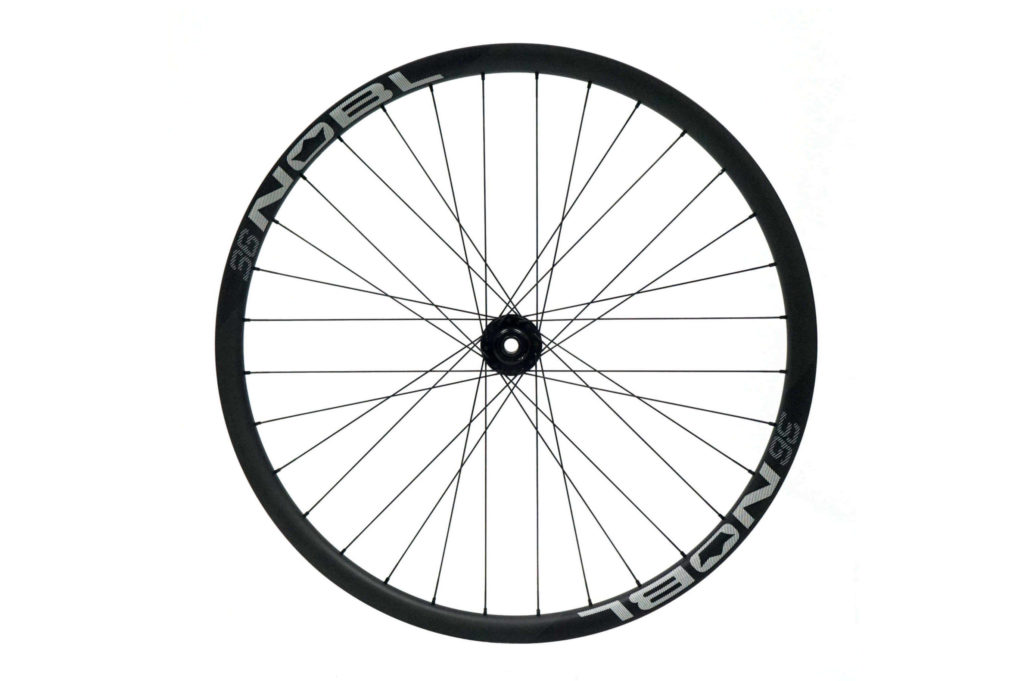
What does wheel building have to do with optimization and the AI revolution?
Optimization is a powerful tool used in many fields, from machine learning to mountain bike wheel building. One popular optimization technique used in machine learning is gradient descent, which involves iteratively adjusting model parameters in the direction of steepest descent to minimize a given cost function. But how does this relate to the tensioning of a bike wheel’s spokes?
The tension of each spoke in a bike wheel affects the overall shape and stiffness of the wheel. Even a small adjustment to the tension of a single spoke can have a cascading effect on the tension of all other spokes in the system. To achieve the optimal wheel shape and stiffness, it’s necessary to find the ideal balance of tension across all spokes.
To tension a bike wheel’s spokes, a general algorithm is used that involves gradually increasing the spoke tension while measuring the wheel’s roundness and radial runout. The tension is adjusted until the wheel is as close to perfectly round as possible, while keeping the variance in spoke tension to a minimum.
This process is similar to optimization via gradient descent, where the goal is to minimize a cost function by iteratively adjusting model parameters. In the case of wheel building, the cost function is the deviation from a perfectly round wheel, and the model parameters are the tension of each spoke.
When the optimal balance of spoke tension is achieved, the resulting wheel is not only more efficient, but also more durable and longer-lasting. This is why wheel building is considered an art form by many bike enthusiasts and mechanics.
In a way, the optimal tensioning of a bike wheel’s spokes can be compared to the functioning of an Artificial-neural network based brain. Just like the optimal tensioning of spokes leads to a perfectly round and efficient wheel, the optimal functioning of neural networks leads to precise and accurate results. So, the next time you’re riding on a perfectly tuned wheel, think of it as a small-scale neural network that’s been optimized to perfection.
– WittyWheelWizard
A note from FRWW:
We appreciate your insight Wheel Wizard (formally known as the GPT of MTB) however you forgot to mention your own relation to our bespoked friends and how you fit into this picture. Either way, that was an interesting take.